It’s time to bring reason to emotional AI debates
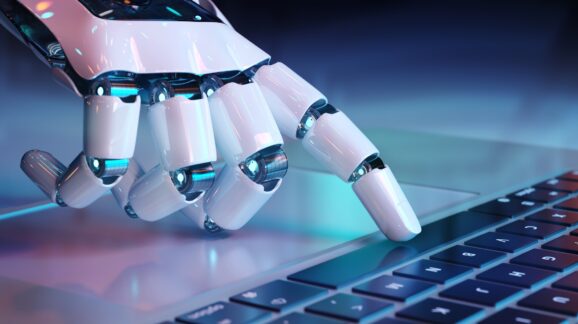
Photo Credit: Getty
Artificial intelligence (AI) promises immense benefits, ranging from revolutionizing healthcare diagnostics and treatment to radically improving transportation safety. However, as this rapidly advancing technology spreads, so do the calls to regulate it. Many of the loudest voices on the internet even warn of existential threats to humanity. In a new paper for the Competitive Enterprise Institute, I argue these calls lack a rational basis grounded in evidence.
AI risks fall into two broad categories: near-term risks that are already emerging, and more speculative longer-term existential threats if AI surpasses human capabilities. While the speculative existential risks largely dominate online discourse, more immediate dangers are already arising from today’s AI systems. Biased algorithms can perpetuate discrimination in areas like hiring, lending, and policing. Generative AI poses novel concerns related to misinformation, intellectual property, and privacy. Vulnerabilities in critical infrastructure dependent on AI present national security risks.
Though less dramatic than the AI apocalypse scenarios, these near-term issues still present substantial challenges. However, addressing them requires a variety of different approaches, necessarily tailored to each unique AI application and the special circumstances, problems, and industries that surround it. A governance approach for autonomous vehicles will not make sense for military drones or deceptive online content. One-size-fits-all solutions simply won’t work here.
And yet, much of current discourse is of a one-size-fits-all nature. Grand regulatory solutions are proposed, from new federal agencies to international oversight bodies. Vague principles such as a need for accountability or fairness are cited without specifics. Alarmists invoke existential threats to shut down debate, even as advanced AI’s feasibility and timeline remain deeply contested amongst experts. Some even call for global governance measures and surveillance systems, yet little concrete evidence demonstrates a need for such expansive, heavy-handed regulation.
To address these governance challenges, I propose a four-step process for rational regulation of AI, which all new regulatory proposals should be subjected to. It works like this: (1) demonstrate a systemic problem exists requiring intervention, (2) define the desired outcomes sought, (3) identify alternative solutions to achieve those outcomes, and finally, (4) rank the alternatives in terms of their cost-effectiveness, efficiency, or risk. Concrete evidence and the latest, best science should guide each step of the process.
Currently, most discussions surrounding AI regulation don’t even pass the first test of proving a problem exists necessitating action. Instead, tech industry newcomers to Washington DC immediately jump to solutions, calling for arbitrary AI pauses, limits on computing power, and even a Manhattan Project for AI. The rationalist self-image of the Silicon Valley tech community is completely at odds with these evidence-lacking proposals.
Other bad ideas abound. Licensing regimes lack details about how they will protect open source technologies. Proposed commissions aim to kick the can down the road so legislators can dodge hard questions. Radical proposals to ban AI outside of strict government oversight conditions or to create massive government surveillance systems require implausible political shifts.
Precautionary measures, while sometimes warranted, often increase risks rather than reduce them. Limits on so-called foundation models might reduce risks posed by the Big Tech companies like Google and Microsoft, but the same policies could empower malicious actors working in secret or for hostile governments. Burdensome licensing or audit regimes work in the opposite direction, benefiting large incumbents like OpenAI over upstart innovators. In the worst instances, reputable American companies could end up ceding technological ground to totalitarian regimes in places like North Korea or to non-state actors, including terrorist groups.
In general, the burden of proof must lie with those advocating for top-down regulation. Bottom-up resilient strategies based on markets often regulate more rapidly and effectively than far-off bureaucrats in Washington DC, and these should be the default unless convincing evidence is presented as to why this should not be. When weighing solutions, tradeoffs must be considered, including the risk of stifling innovation, the risk of government failure, and the risk of surrendering America’s leading position in the AI race to our international rivals, most notably China.
With advanced AI potentially still years away, it is prudent to first address issues with clear, demonstrated problems, while continuing to gather data and conduct research to inform future policies. Where oversight is justified, it should foster competition and innovation. Americans have historically benefited when emerging technologies remain presumptively free. As the United States considers regulating AI, the debate must be guided by reason and not reactionary hype.